Abbrev | Film | Clip Start | Clip Stop | Duration |
---|---|---|---|---|
LittleMiss | Little Miss Sunshine (2006) | 00:13:10.000 | 00:15:20.000 | 130 |
Little Miss Sunshine
Film Information
All information in this section came from Wikipedia.
Clip Information
This scene shows a conversation at the dinner table. Frank recently came to live with the family of his sister, Sheryl, after attempting suicide. At the table are Sheryl, her brother Frank, her husband Richard, her children Dwayne and Olive, and Richard’s father Edwin.
Characteristic | Value |
---|---|
Format | MPEG-4 |
File Size | 91.5 MiB |
Duration | 130.005 |
Frame Rate | 23.976 |
Video Width | 1920 |
Video Height | 796 |
Video BitRate | 5.6 MB/s |
Audio Channels | 6 |
Audio SamplingRate | 48000 |
Audio BitRate | 341.1 kB/s |
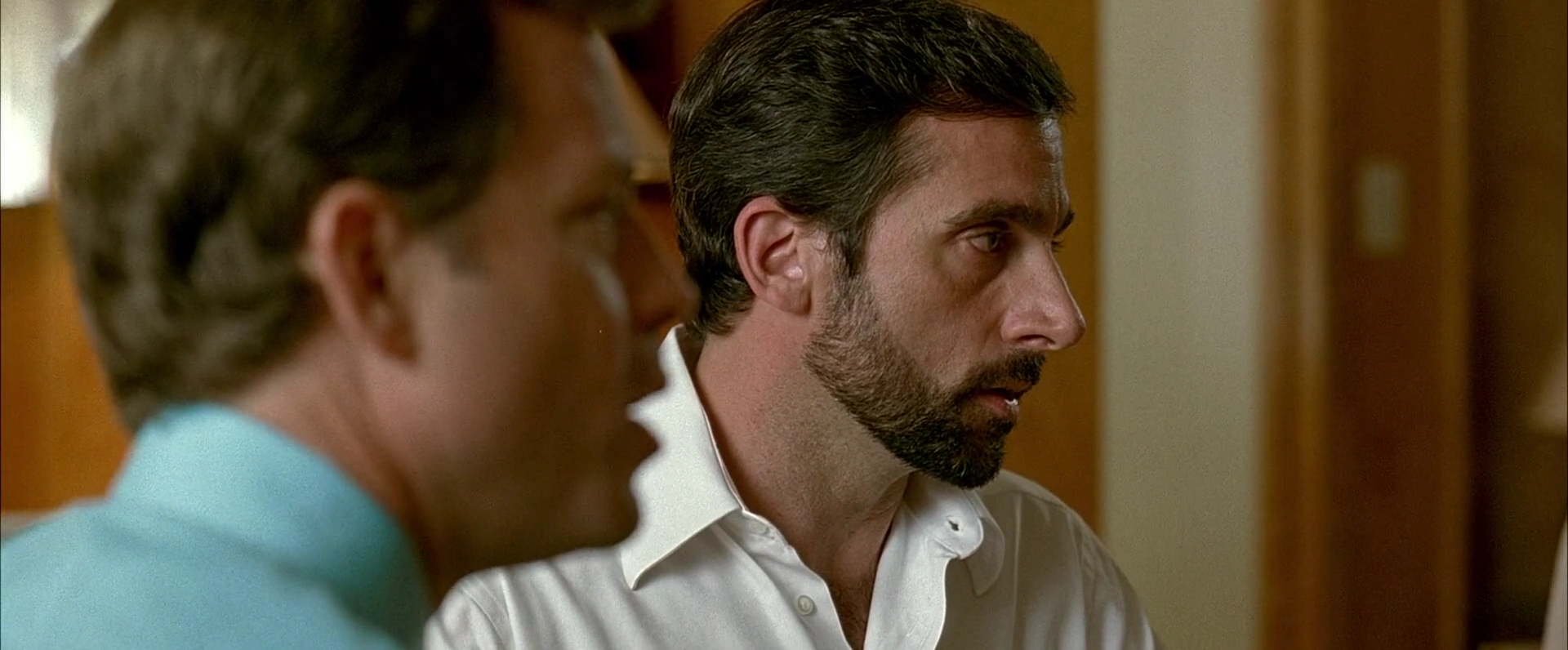
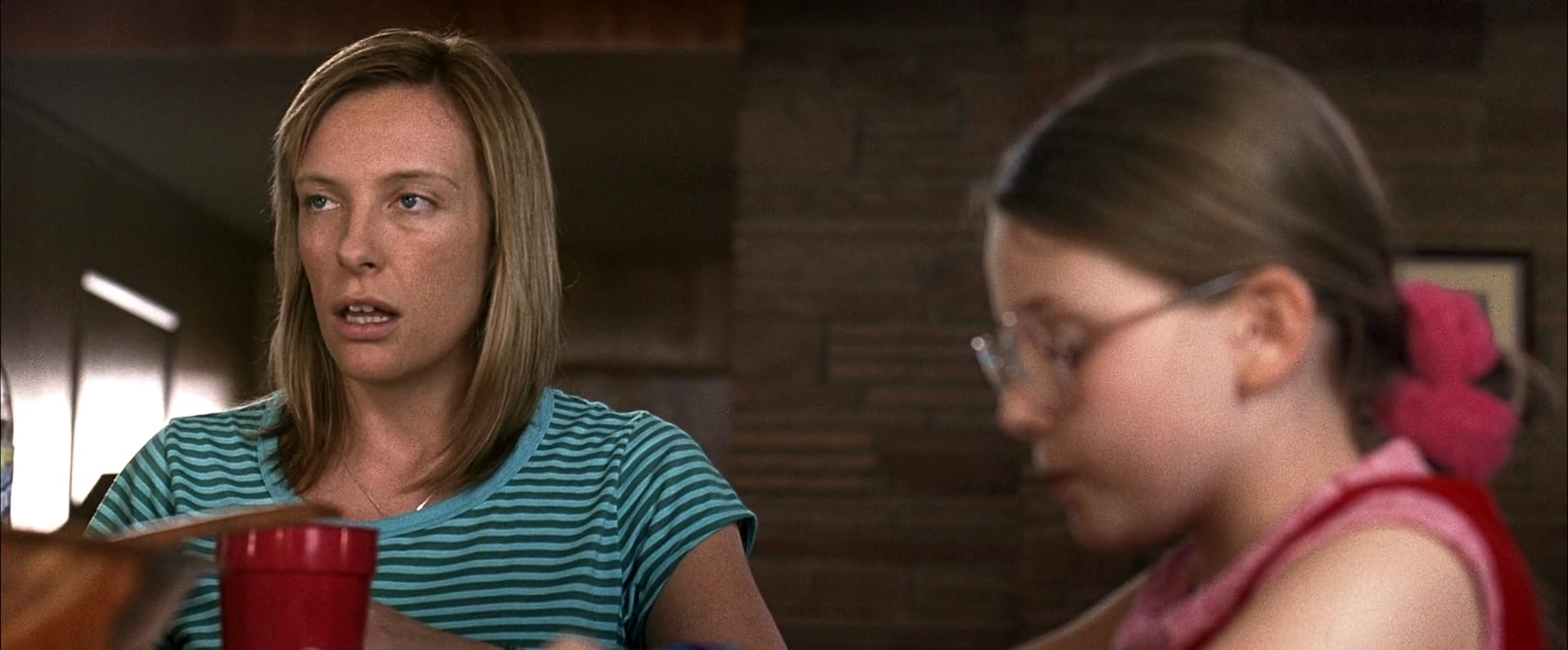
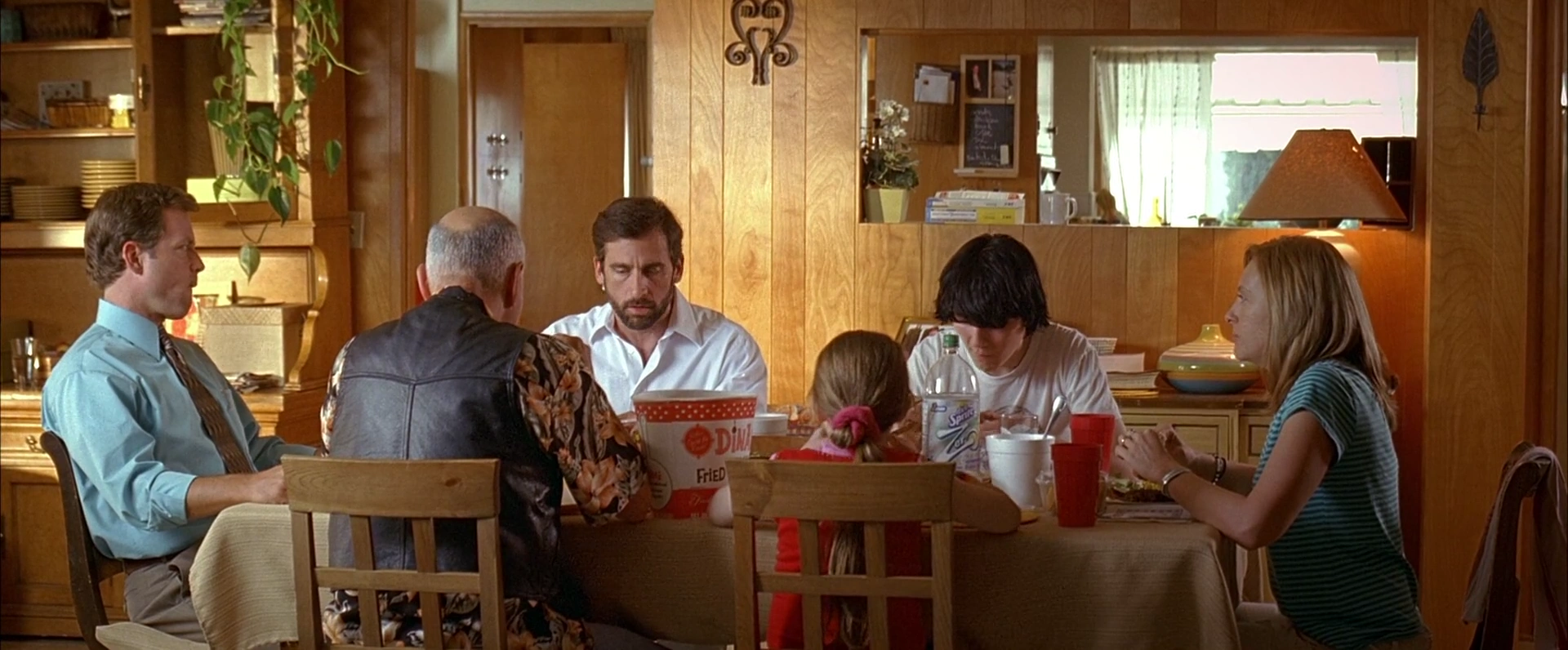
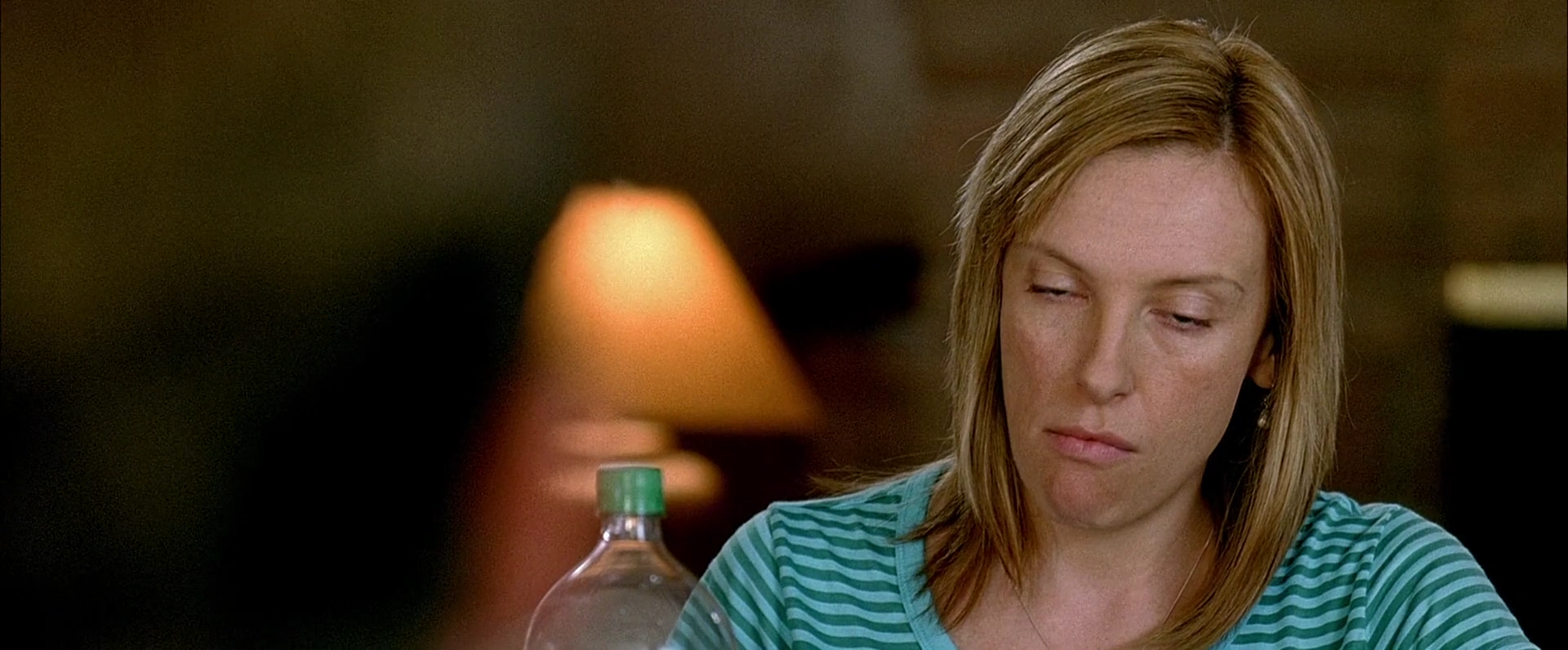
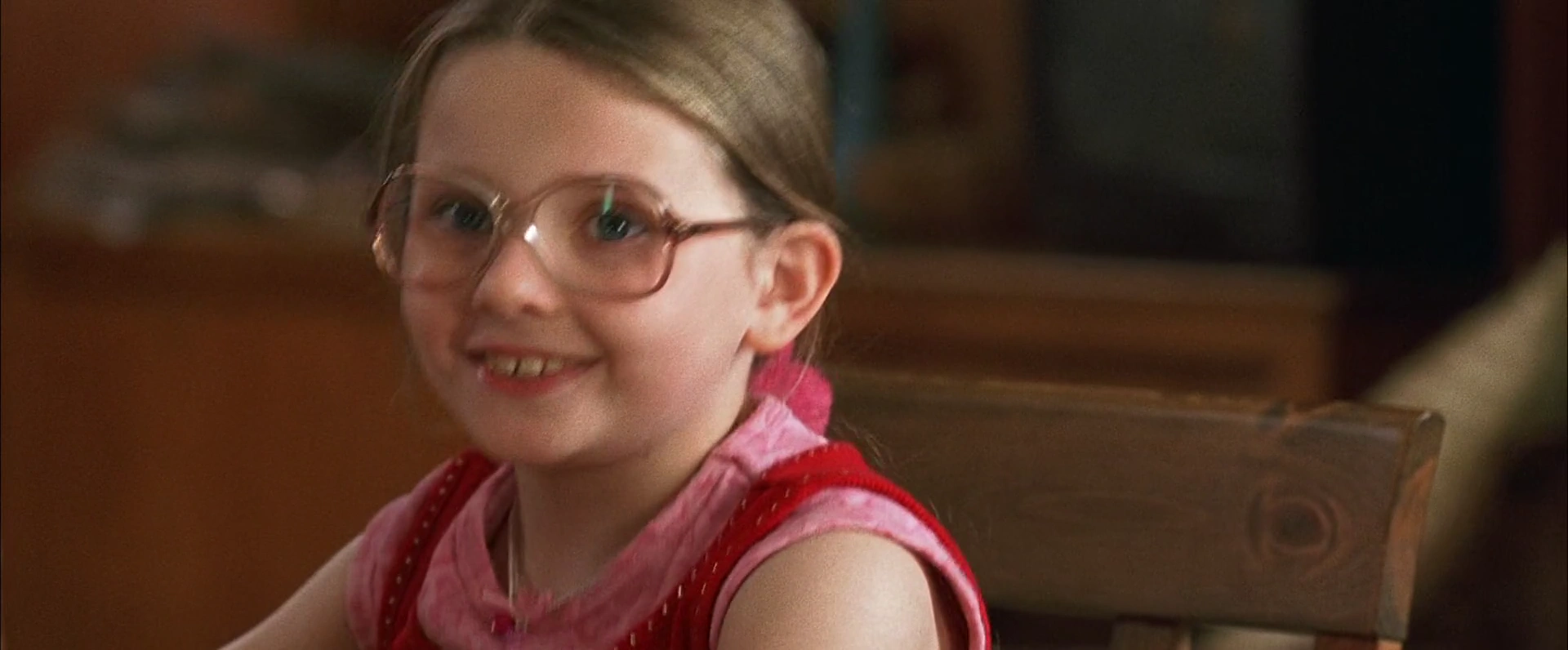
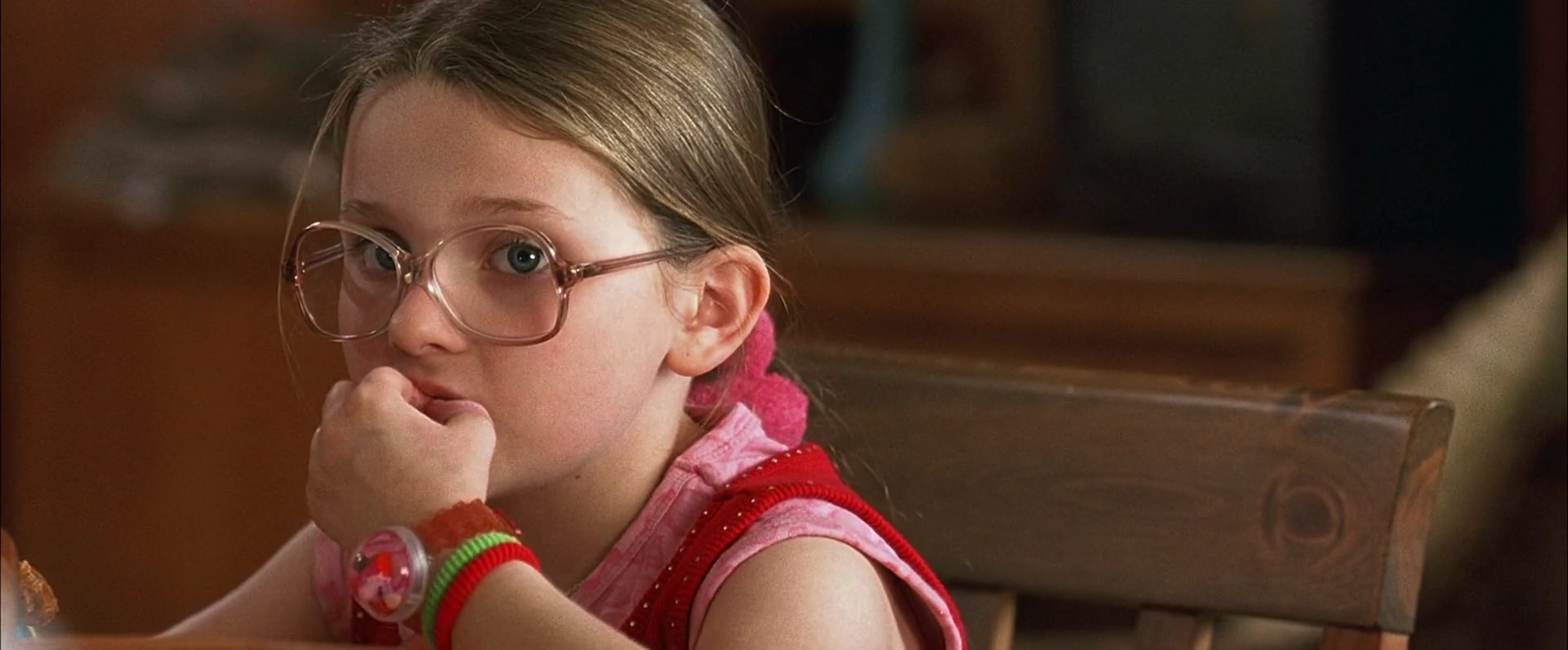
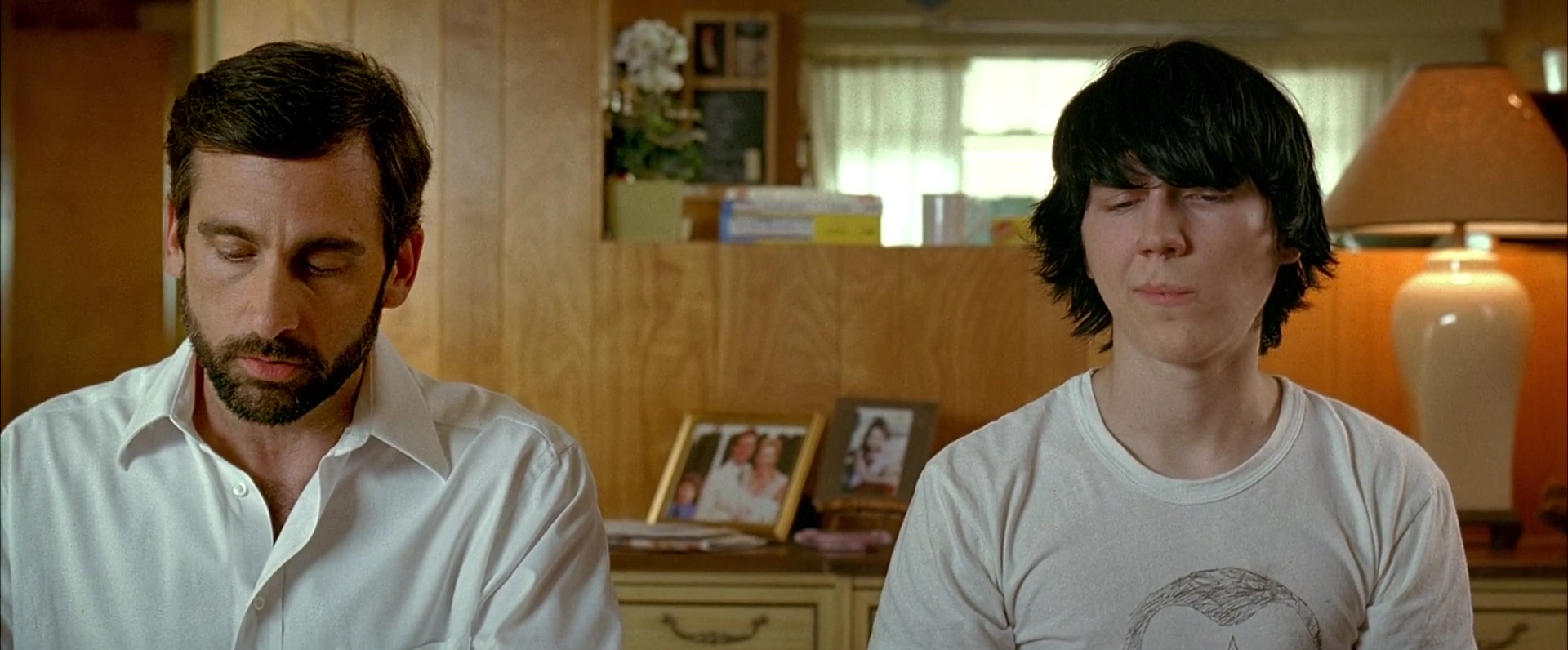
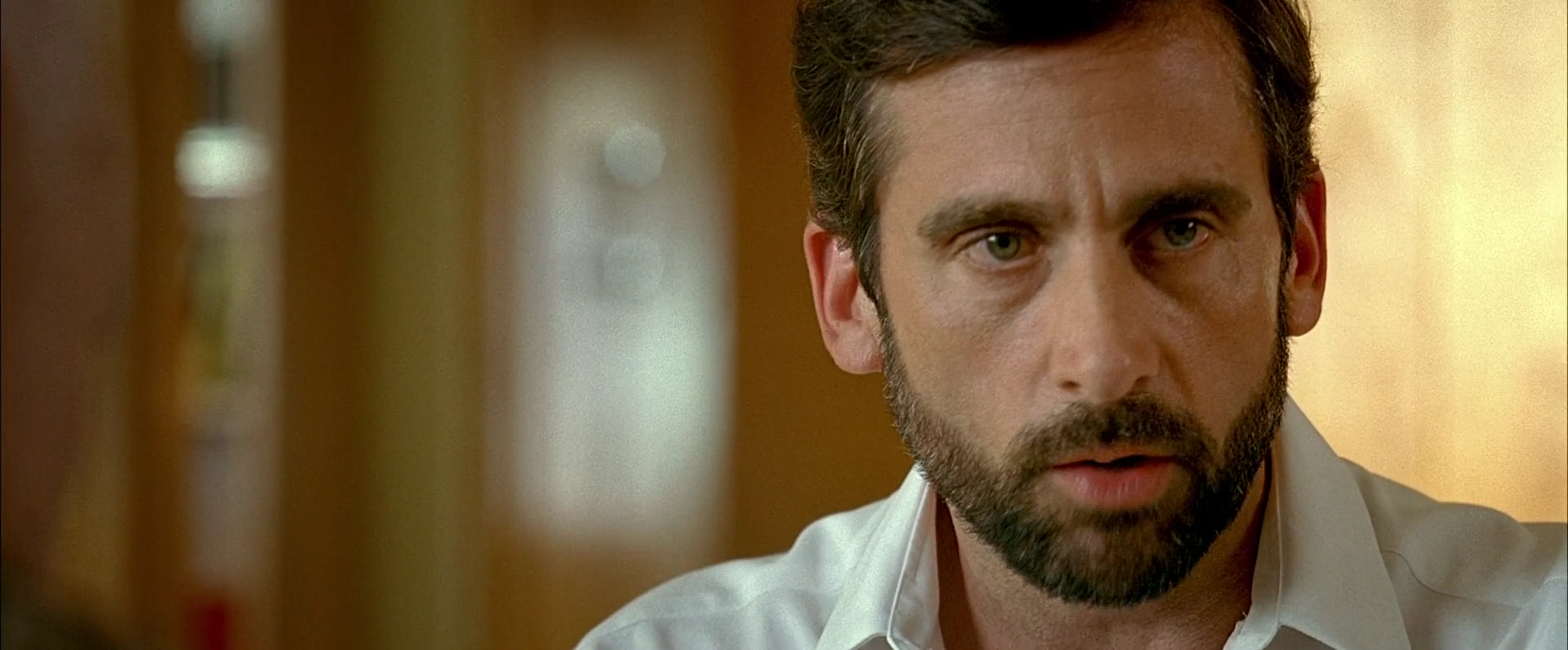
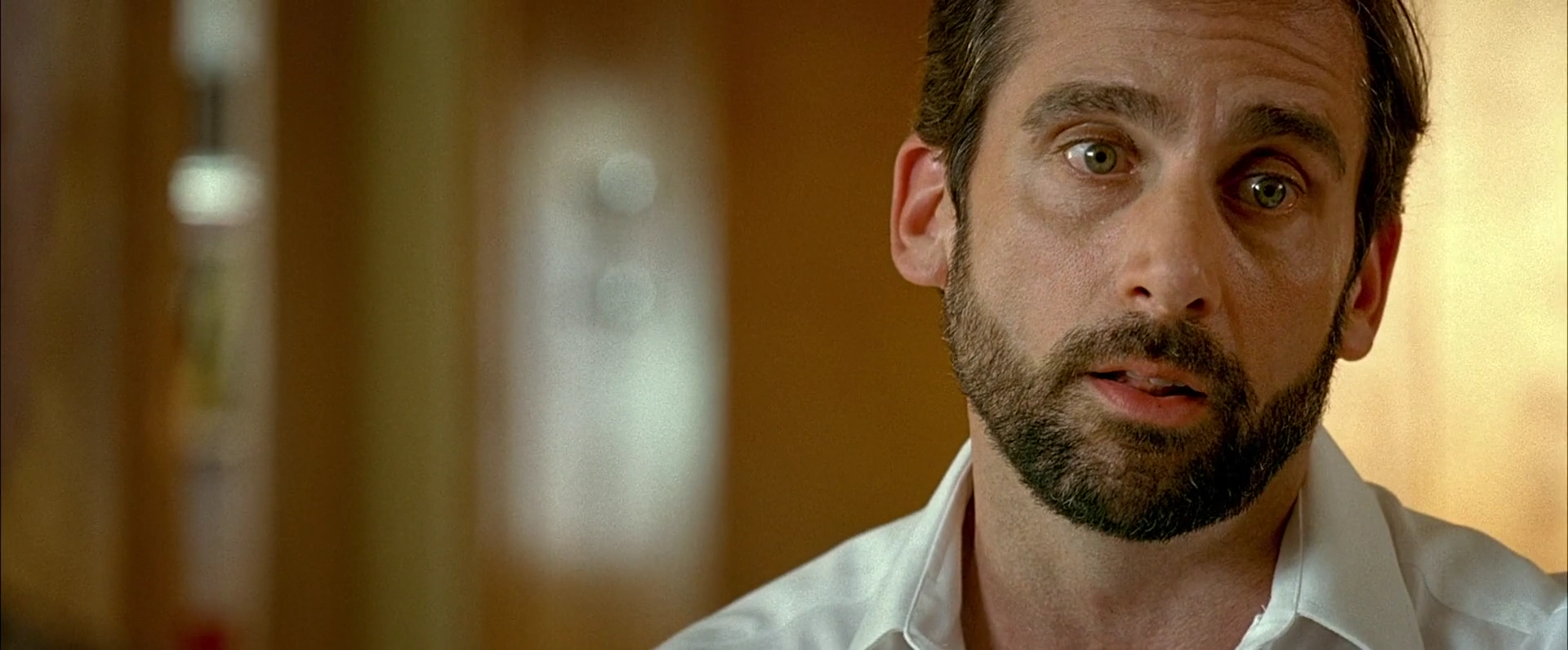
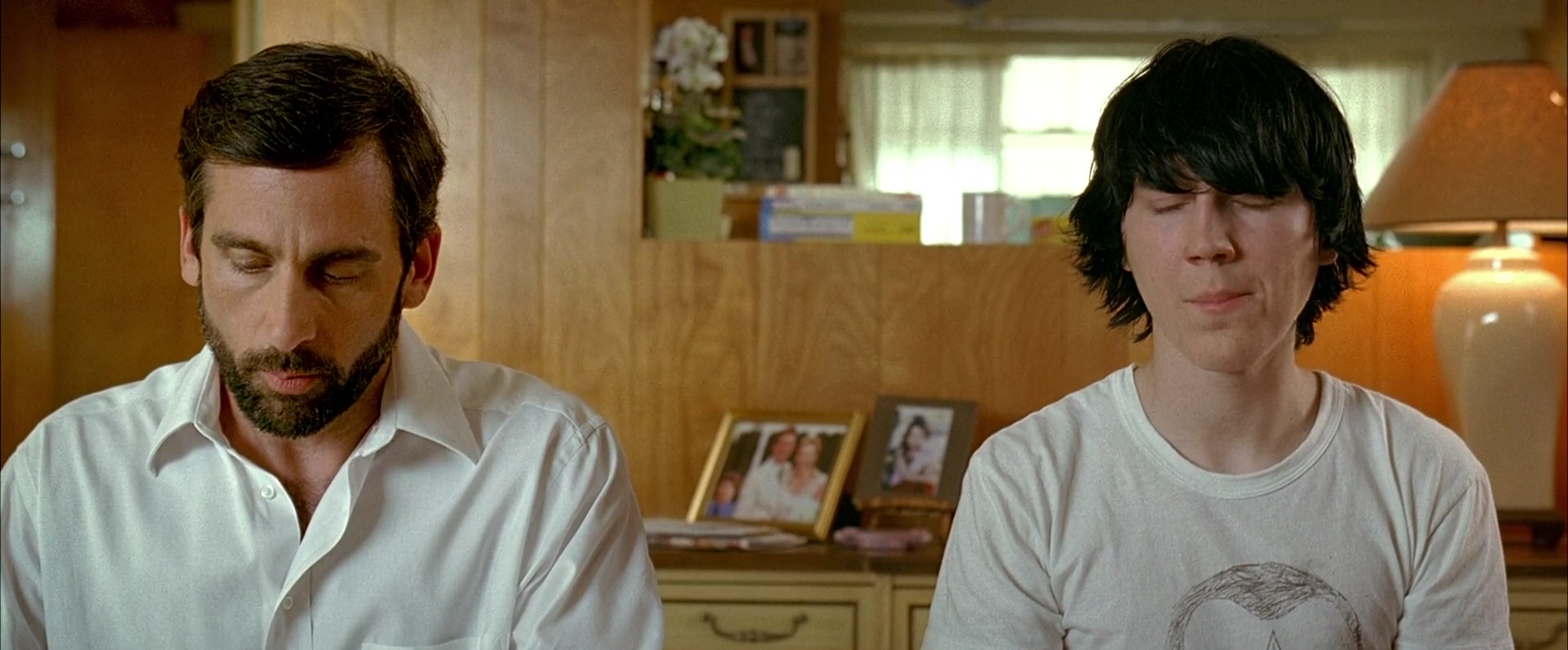
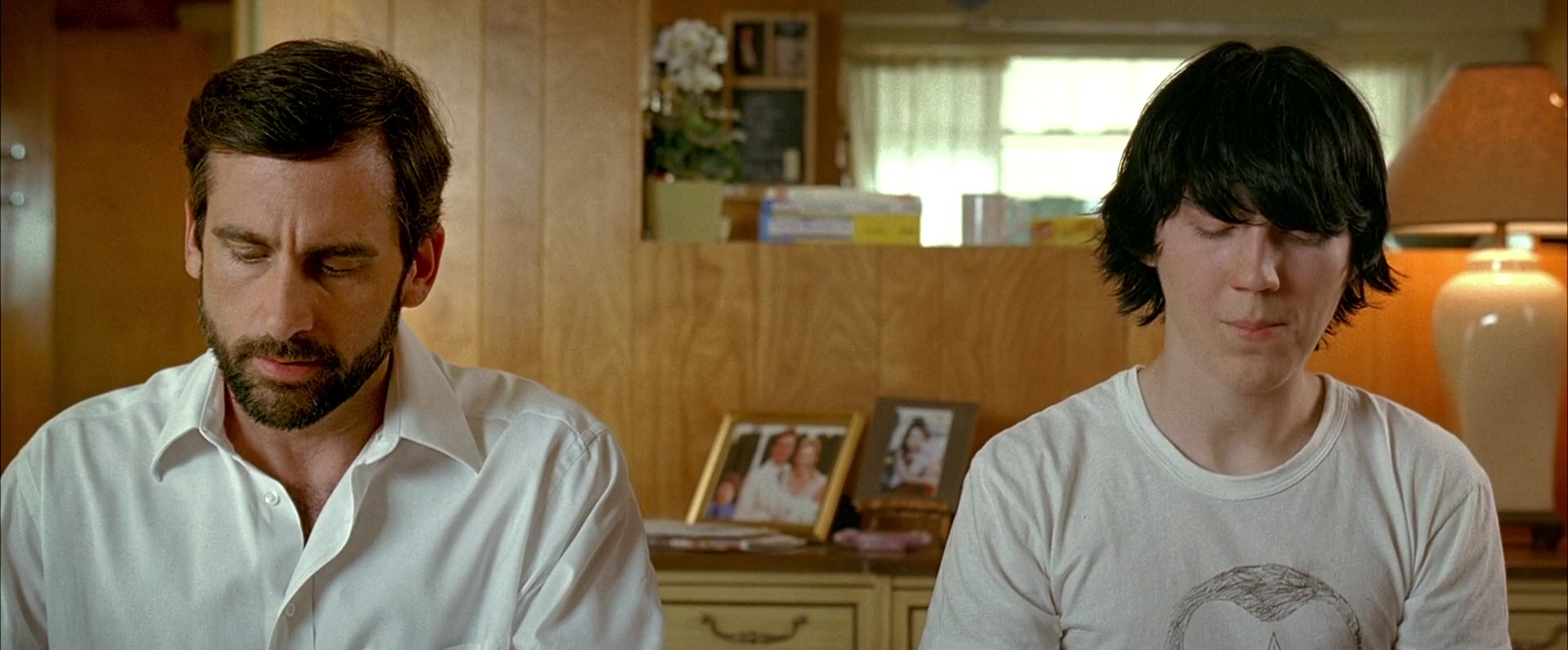
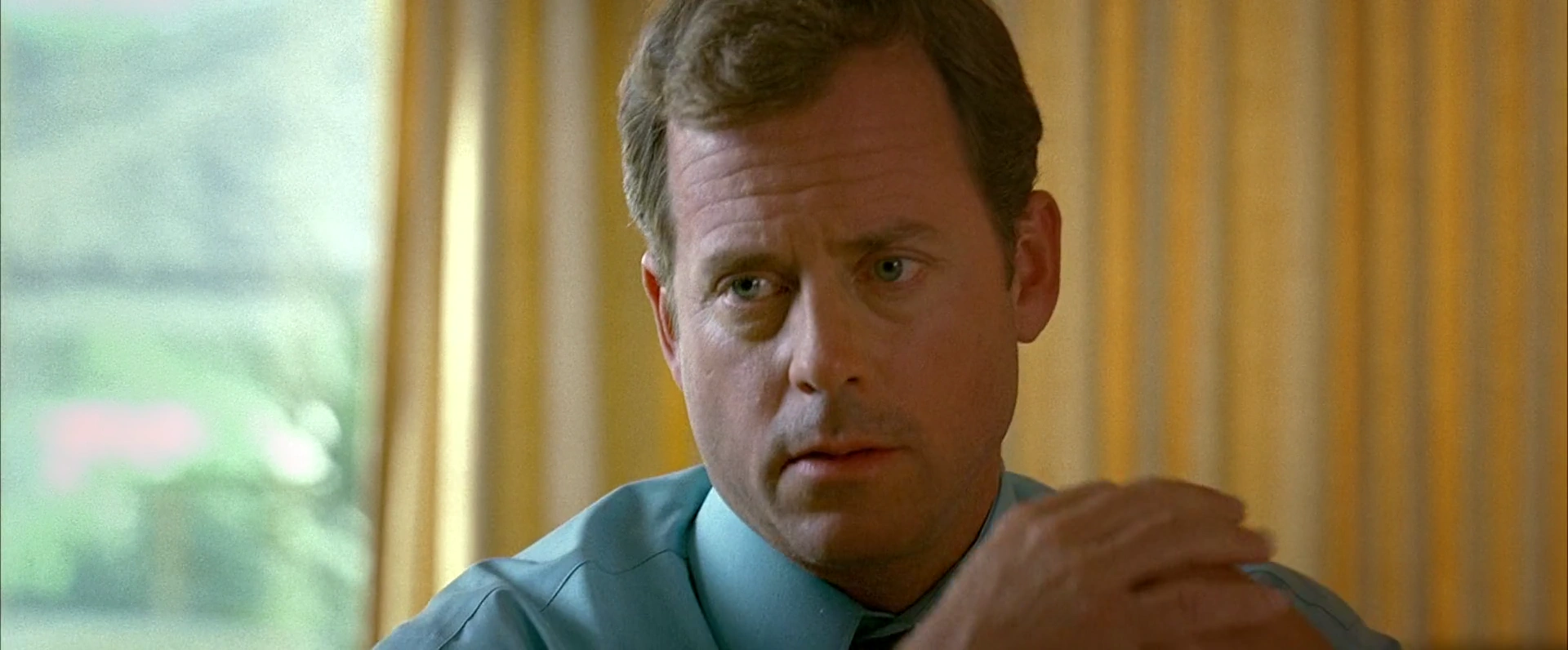
Subtitles
The following wordcloud shows the words used in this clip, scaled by number of occurrences and colored by sentiment (orange = negative, green = positive, grey = neutral or unsure). Note that the words have been stemmed and lemmatized and stopwords have been removed.
The table below shows all subtitles in this clip with the start and stop time of each subtitle’s appearance in seconds.
Start | End | Subtitle |
---|---|---|
0.000 | 2.395 | Why did you want to kill yourself? |
2.495 | 4.994 | No, don't answer the question, Frank. |
5.104 | 7.534 | - Richard! Richard! - He's not gonna answer the question. Frank. |
7.634 | 10.074 | - I wanted to kill myself- - Don't listen to him. |
10.174 | 12.304 | - I was very unhappy. - He's sick in his head. |
12.404 | 14.804 | - Richard! - I'm sorry! I don't think it's an appropriate conversation... |
14.914 | 17.074 | - for a seven-year-old. - She's gonna find out anyway. |
17.184 | 21.084 | - Okay. - Go on, Frank. |
24.284 | 26.514 | Why were you unhappy? |
26.624 | 30.084 | Um, well, there are a lot of reasons. |
30.194 | 35.103 | Mainly, though, I fell in love with someone who didn't love me back. |
35.203 | 36.763 | Who? |
36.873 | 40.103 | One of my grad students. I was very much in love with him. |
40.213 | 42.583 | Him? |
42.683 | 47.013 | It was a boy? You fell in love with a boy? |
47.123 | 50.243 | - Yes, I did. Very much so. - That's silly. |
50.353 | 53.053 | You're right. It was silly. It was very, very silly. |
53.163 | 56.223 | - There's another word for it. - Dad. |
56.333 | 60.233 | - So, that's when you tried to kill yourself? - Well, no. |
60.333 | 63.892 | The boy that I was in love with fell in love with another man- Larry Sugarman. |
64.002 | 66.262 | - Who's Larry Sugarman? - Larry Sugarman is, perhaps... |
66.372 | 69.532 | the second most highly regarded Proust scholar in the U.S. |
69.642 | 73.202 | - Who's number one? - That would be me, Rich. |
73.312 | 75.712 | - Really? - Mm-hmm. |
75.812 | 77.942 | So that's when. |
78.052 | 80.412 | No. What happened was I was a bit upset... |
80.522 | 82.812 | so I said some things that I shouldn't have said... |
82.922 | 85.052 | and I did some things that I shouldn't have done... |
85.152 | 87.212 | and subsequently I was fired from my job... |
87.322 | 90.491 | and forced to move out of my apartment and move into a motel. |
94.261 | 96.751 | - And that's when you tried to- - Well, no. |
96.871 | 99.061 | Actually, all of that was okay. |
99.171 | 103.761 | What happened was two days ago the MacArthur Foundation, in its infinite wisdom... |
103.871 | 108.071 | awarded a genius grant to Larry Sugarman. |
108.181 | 110.441 | And that's when I- |
110.551 | 113.141 | Decided to check out early. |
113.251 | 116.811 | Yes. Yes. And I failed at that as well. |
116.921 | 120.910 | Olive, the important thing to understand here... |
121.020 | 123.250 | is that Uncle Frank gave up on himself. |
123.360 | 127.290 | He made a series of foolish choices- I'm sorry- and he gave up on himself... |
127.400 | 129.960 | which is something winners never do. |
Holistic Ratings
A total of 78 participants watched this film clip and then provided holistic ratings on how the entire clip made them feel. These holistic ratings were completed using five Positive Affect items (i.e., alert, determined, enthusiastic, excited, inspired) and five Negative Affect items (i.e., afraid, distressed, nervous, scared, upset), each rated on an ordinal scale from 0 to 4. The plot below shows the
Dynamic Ratings
A total of 75 participants watched this film clip and used the CARMA software to provide continuous (i.e., second-by-second) ratings of how it made them feel. These continuous ratings were made on a single emotional valence scale ranging from -4 (very negative) to 4 (very positive).
Time Series
We can plot the distribution of all valence ratings per second of the film clip to get a sense of how its emotional tone changes over time. The solid black line represents the mean of all ratings and the yellow, green, and purple ribbons represent the central 50%, 70%, and 90% of the ratings, respectively.
Inter-Rater Reliability
A Bayesian generalizability study was used to decompose the variance in ratings of this video clip into the following components: timepoint variance (in average ratings of each second, across raters), rater variance (in average ratings from each rater, across seconds), and residual variance (including second-by-rater interactions and measurement error). The lower and upper columns in the table below represent the boundaries of the 95% equal-tail credible interval. Note that we dropped the first 10 seconds of each clip (as rater “warmup” time).
Component | Term | Estimate | Lower | Upper | Percent |
---|---|---|---|---|---|
Rater | Variance | 1.547 | 1.183 | 2.275 | 0.704 |
Timepoint | Variance | 0.071 | 0.057 | 0.101 | 0.032 |
Residual | Variance | 0.578 | 0.561 | 0.596 | 0.263 |
From these variance components, we can estimate inter-rater reliability of the ratings. There are many formulations of the two-way intraclass correlation (ICC), but the most relevant to our purposes here is the balanced average-measures consistency formulation or ICC(C,k).
Term | Estimate | Lower | Upper | Raters | Error |
---|---|---|---|---|---|
ICC(C,k) | 0.91 | 0.881 | 0.929 | 75 | Relative |
Below, we can also visualize the posterior distributions of each of these parameters. Values with higher posterior density are more probable.